An AI That Sees Bird Futures
Using citizen data to predict where the birds go
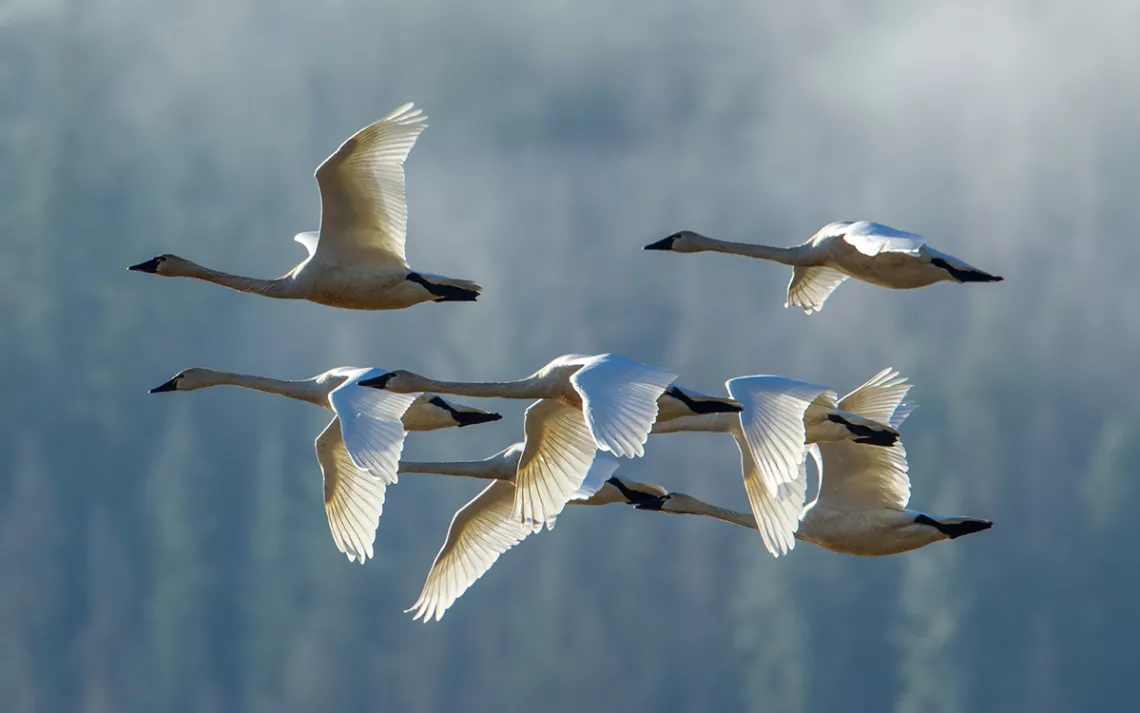
Tundra swans in formation. | Photo by gjohnstonphoto/iStock
Every day, scientists and birders across the world log hundreds of thousands of bird sightings into eBird, an online database run by the Cornell Lab of Ornithology. The data, which has been accumulating since 2002, is used by scientists to track changes in bird distribution and to know where a specific species is at any given time.
But there was always a “piece missing,” said Cornell ornithologist Benjamin Van Doren. Ebird could only show where birds had been seen, not where they were going. Last month, Van Doren and a team of other researchers at Cornell and the University of Massachusetts, Amherst, announced that BirdFlow, a new “probabilistic modeling framework” they’d developed, was able to predict the likely position of a bird species several weeks into the future, once it was given a starting time and location. The group used BirdFlow to predict the movements of 11 species of birds, checking those predictions against data collected from birds that were equipped with trackers.
A phrase that people often use as shorthand for “probabilistic modeling network” (as well as a variety of other tools that use computer modeling and statistics) is “artificial intelligence” or AI. Is BirdFlow AI? According to Daniel Sheldon, a computer scientist at the University of Massachusetts, Amherst, and one of the paper’s authors, absolutely—though, he said, AI is an umbrella term for many different computational tools.
BirdFlow makes its predictions by using two main factors: information about the biological cost of migration and distribution maps generated from eBird. The maps are gridded into tiny cells, like a board game, and BirdFlow uses the data that it has to try to predict the likelihood that a bird will land on each cell. Like other AI systems, BirdFlow “learns”—that is, it is able to adjust its predictions based on comparisons with the actual routes birds take. To Sheldon, this is what makes BirdFlow an AI—it gets better at deducing the most accurate migration routes from the available data.
The end result is a map that can show the likely future locations of a given bird, within a range of about 80 to 150 miles (some species are harder to predict than others). Kyle Horton, an assistant professor of ecology at Colorado State University, was not involved in the creation of BirdFlow and described it as a “significant improvement” to the existing models available to scientists. “The toolbox for studying migration is limited,” he said.
Van Doren thinks that BirdFlow could be used to help save habitat for migratory birds. There are wide swaths of North America where birders rarely tread, and scientists still don’t have clear data on where some species of endangered birds go during certain phases of their life cycle. This information shortfall impacts migratory connectivity—the ability of the birds to have healthy habitats in every stage of their migration. Sheldon thinks that BirdFlow could someday be used to help limit the spread of diseases, such as avian influenza, by predicting where infected wild birds might move.
Van Doren emphasized that BirdFlow is still in its early stages. Even its applications in conservation are still “a little ways off”—for now, he expects BirdFlow to be primarily a research tool. Meanwhile, a growing number of other artificial intelligence tools are being developed for conservation work. PAWS, developed by Carnegie Mellon researchers, generates suggested poaching patrol routes for law enforcement. TrailGuard AI claims to deter wildlife poaching by identifying nearby human visitors. Recently, said Sheldon, there has also been a big push to use AI to recognize organisms in photos, audio, and video so that scientists can automatically monitor ecosystems. Cornell’s Merlin birding app uses AI to recognize bird species based on their songs and their images.
Some scientists outside of North America and Europe have criticized eBird and databases like it for their focus on birdwatching as the primary method of data collection, arguing that in locations outside the US particularly, eBird data tends to be generated by tourists and other outsiders, rather than locals. BirdFlow’s creators acknowledge that it probably has limitations—two flocks of birds may be the same species, for example, but make very different decisions while migrating. Sheldon also said the team is trying to figure out how to incorporate data from sources beyond eBird, such as bird location data from satellite or GPS tracking.
But Sheldon also sees this merging of computer science and conservation as critical for challenges like slowing biodiversity loss. In a 2019 paper titled Computational Sustainability: Computing for a Better World and a Sustainable Future, Sheldon and 28 other scientists argued that “computer scientists … should play a key role in helping address societal and environmental challenges in pursuit of a sustainable future.” Asking how AI will change conservation is like asking how statistics inform conservation, said Van Doren. Instead, “it is proliferating every aspect of scientific inquiry.”